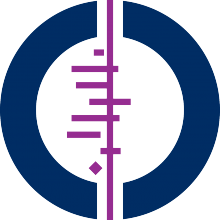
Cochrane’s iconic logo is actually a forest plot from a hugely influential systematic review.
Today’s post is a short one. The idea emerged as I read through the tireless blogging from David Gorski and others and the work of epidemiologists like Gideon Meyerowitz-Katz who have painstakingly gone through the ivermectin literature, trial-by-trial, even scrutinizing patient-level data, to uncover some truly poor science (some of which appears fraudulent). Through their work, they’ve exposed how research studies are being misrepresented, even weaponized, to promote the use of ivermectin.
This post is not about ivermectin. It is about the tools we use to bring some sense to the medical literature – and ways in which casual readers, and even seasoned professionals, can be misled. I want to summarize some of the methods used to compile and summarize evidence, and the approaches that can be used reduce biases and improve the quality of our conclusions and decisions about our health.
Finding good evidence
To guide decision-making in medicine, we need effective methods to identify, compile, and analyze volumes of information. The amount of evidence that’s generated on a daily basis is near-impossible for anyone to keep up with, even if the specific topic you’re interested in is niche or narrow. New evidence can be unclear and even contradictory, and the “best evidence” can change quickly. Just look at how the pandemic guidance has changed since early 2020, from the utility of masks, the relevance of handwashing, and how to manage (what is now recognized as) an airborne infection.
Let’s say you’re interested in therapies to treat COVID-19 infections. The World Health Organization has cataloged over 334,000 publications in its COVID-19 database. If I restrict my search just to clinical trials for COVID-19 treatments, I’m still left with over 1,000 papers, with more emerging daily. How do we identify the high quality, most relevant evidence from weaker evidence – and how should we try to wrap our head around the totality of all of this data?
A single clinical trial is usually insufficient (though not impossible) to change clinical practice. That’s often because there are so many considerations when asking a specific clinical question and designing a trial. What dose? What patient population? What is the treatment protocol? What is the endpoint that’s being measured? How large was the trial? And was the trial conducted in a way to be a truly “fair test” of a treatment – meaning blinding and appropriate controls? Several trials, may be conducted by different investigators, with a mix of findings. What’s needed is a method to identify the best of the best, and to try to synthesize what may appear to be contradictory findings.
In a lot of cases, we rely on the work of others to give us that perspective. Narrative reviews (like a blog post!) are the quickest and easiest, but the most prone to bias, because the author can cherry-pick the evidence to comment on:
A narrative review tends to be a discussion of a topic, where the author decides what evidence is relevant and worth discussing. They may be written by experts who have a deep understanding of the topic, and will summarize pertinent data and make general conclusions. This is not to say that narrative reviews are necessarily inaccurate – only that they can be prone to biases that may not be easy to spot. A narrative review leaves the reader totally in the hands of the author. For that reason, reading one or more narrative reviews can be reasonable starting points to understanding a topic, but cannot be considered comprehensive or definitive summaries of the evidence base of a topic or clinical question.
Systematic reviews and meta-analyses
Sometimes we want to know what the best evidence says. The opposite of the narrative review is the systematic review. That’s where the evidence is formally and systematically searched and analyzed.
A systematic review is a summary of the evidence that addresses a specific question and uses explicit, rigorous, and transparent methods to identify, select, appraise, and combine research studies. The included trials must be identified from a systematic search, with pre-defined quality criteria, that should be published in advance of the search itself. Researchers will identify the search strategy, the selection criteria (which trials will be included) and the analytic methods that will be applied. Doing all this in advance reduces the risk of bias when the data is actually analyzed. PRISMA (Preferred Reporting Items for Systematic Reviews and Meta-Analyses) defines the minimum data requirements that should be reported in a systematic review, improving the quality, consistency, and transparency of reviews.
A meta-analysis can be part of a systematic review, but can also be done on its own. (A systematic review appraises data before it is combined and analyzed in a meta-analysis.) It is a statistical compilation of multiple studies that examine the same topic, and it can be a useful tool as part of a systematic review. Done carefully, then can give a “point estimate” (aka “best guess”) of an effect that may be more precise than any of the individual trials that were included. But just as individual clinical trials can be biased, so can meta-analyses. Meta-analyses cannot correct for fundamentally poor source data. That is, they cannot glean accurate information from flawed research. The “garbage in, garbage out” adage applies here. Moreover, if different trials measured different endpoints, then they cannot be combined in a meta-analysis. Or you’ll see something kind of like this:
This is statistical heterogeneity, as discussed in a post by Dr. William Paolo back in August:
When analyzing the results of a meta-analysis it is imperative to focus on the degree of statistical heterogeneity to determine if the resultant point estimate of the compiled studies are, in fact, similar enough to be combined into a singular parsimonious estimate of effect. Theoretically, multiple smaller studies can be pooled and analyzed as a singular body of evidence if the component studies are of analogous design and methodology (allocation concealment, randomization, intention to treat analysis etc.) or rejected if this is not the case. It is required that the included studies be the same in terms of design, inclusion and exclusion criteria, demographics, equivalent doses of an intervention, outcomes, and a myriad of other factors to comfortably combine said studies into a unitary body of evidence. Concordant with this is the expectation that if the included studies of a meta-analysis are of equal kind that experimentally derived results, taken under similar conditions, should roughly approximate one another. In other words, all things being equal, the same exact experiment done in multiple places by different people should roughly find the same results if the studies are in fact similar enough to be analyzed as one.
Ivermectin trial alchemy
With the above in mind, let’s take a closer look at how the meta-analysis has been misused in the pandemic. Ivmmeta.com is a site that is frequently cited by advocates of ivermectin which, as David Gorski first discussed way back in June, seemed to brought to us by the people that created hcqmeta.com (Remember hydroxychloroquine? How vintage.) It’s impressive, in an information-overload kind of way, claiming to be a “real-time meta-analysis of 67 studies”. Rather than go through the criticisms of the website in detail, I will point you to the writing and blogging of Gideon Meyerowitz-Katz who has scrutinized the data presented – and the website’s response to his initial criticisms.
In something of a hilarious development, the ivmmeta authors have added a section titled "with GMK exclusions" to their website
Unfortunately, this still includes endless awful studies. Let's take a look at some of the papers that remain on this website https://t.co/moT5tNnD1n
— Health Nerd (@GidMK) October 26, 2021
In short, ivmmeta.com (or sister sites, like c19ivermectin.com) does not adequately take into account the quality and diversity of the data it is combining. As David Gorski highlighted, there are warnings in the actual literature about this:
Different websites (such as https://ivmmeta.com/, https://c19ivermectin.com/, https://tratamientotemprano.org/estudios-ivermectina/, among others) have conducted meta-analyses with ivermectin studies, showing unpublished colourful forest plots which rapidly gained public acknowledgement and were disseminated via social media, without following any methodological or report guidelines. These websites do not include protocol registration with methods, search strategies, inclusion criteria, quality assessment of the included studies nor the certainty of the evidence of the pooled estimates. Prospective registration of systematic reviews with or without meta-analysis protocols is a key feature for providing transparency in the review process and ensuring protection against reporting biases, by revealing differences between the methods or outcomes reported in the published review and those planned in the registered protocol. These websites show pooled estimates suggesting significant benefits with ivermectin, which has resulted in confusion for clinicians, patients and even decision-makers. This is usually a problem when performing meta-analyses which are not based in rigorous systematic reviews, often leading to spread spurious or fallacious findings.36
You might be asking at this point – has anyone actually done a robust systematic review of ivermectin? The answer is yes, and the results will not surprise (spoiler: ivermectin doesn’t seem to work).
A tool, not a quality indicator
All types of data can be combined into a meta-analysis. When used to study high-quality trials of similar populations that used similar methods, they can be powerful tools. But a meta-analysis is just a tool, and cannot make gold out of lead. Combining poor quality studies that are not sufficiently similar in a meta-analysis does not give us any useful information. Moreover, in the absence of rigorous, transparent, and pre-specified rules, meta-analyses are prone to bias, just like the source clinical trials themselves. Meta-analyses prepared outside the framework of a rigorous systematic review should be viewed with caution.